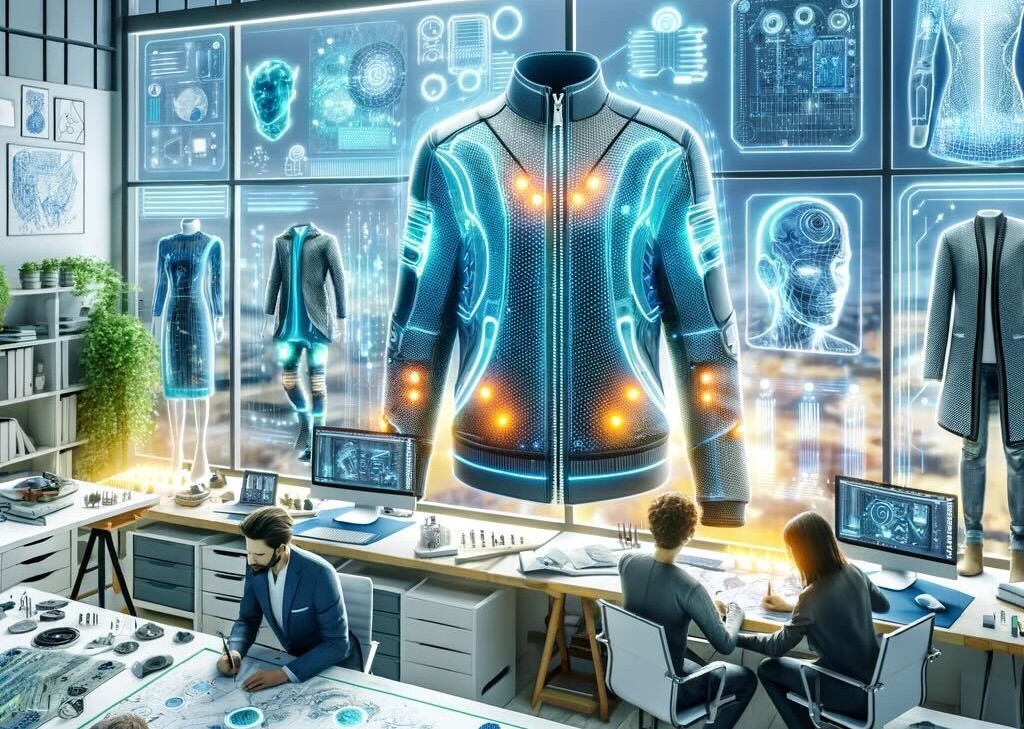
Editor’s note: Two researchers with expertise in advanced technologies—and smart textiles, in particular—responded to questions about artificial intelligence and its future in the industry. Irmandy Wicaksono (IW) is a research assistant in the MIT Media Lab and lead author of a paper presenting 3DKnITS. Caitlin Knowles, Ph.D., (CK) is an e-textile device engineer with the Advanced Functional Fabrics of America (AFFOA).
Q: I’m interested in where the intersect of smart materials and artificial intelligence can, or is most likely, to happen. What can AI offer smart materials that can’t be provided with current (or developing) technologies?
IW: Smart materials integrated with sensing and computational capabilities are paving the way for advanced textiles and garments including those that can seamlessly and wirelessly monitor our physiology and physical activity. Our research on the Electronic Textile Conformable Suit (E-TeCS) exemplifies this direction: to change the way we do healthcare from curing to preventing and constant care, demonstrating its application for telemedicine. Leveraging AI will help us make sense of and recognize patterns from the extensive and continuous “big data” captured from these smart garments’ multimodal sensors, thereby advancing health and sports analytics.
We have recently demonstrated the fusion of AI and smart textiles in our work on the 3DKnITS project, where we utilize deep learning—typically employed in image and audio processing—to analyze a large dataset of pressure images from our smart mat and shoes for real-time recognition of body postures, exercises and activities. As we gather more data at individual, collective and environmental scale through these ubiquitous smart materials, the importance of AI in processing this complex data and facilitating interventions will become clearer.
Q: We know that larger success for smart textile products has been stalled by how best to power them, and to make the power source “seamlessly” integrated into the product itself. Is there a role to play for AI in solving this problem?
CK: AI can absolutely aid in e-textile integration strategies, and with e-textile design more generally. AI tools can generate patterns that could be optimized for certain objectives such as fit, material use or waste reduction. This could theoretically be extended to e-textile routing and component placement.
One of the challenges in e-textile design is that prototypes can be costly and time consuming to create, and there are a seemingly infinite number of material, structure, and integration strategies to explore and down select. AI, armed with a well-defined set of constraints and objectives and plenty of prior data to reference, could assist in decision making by rapidly evaluating a large number of possible combinations and outputting the most promising. Of course, the effectiveness of these tools relies on the quantity and quality of data that the models are trained on.
IW: We have seen several efforts in developing miniaturized or fiber-like rechargeable batteries and energy harvesters that can be integrated into fabrics through knitting, weaving, or screen printing, with solar, kinetic and thermoelectric sources emerging as viable power scavenging options. AI-informed computational design could assist in selecting the optimal materials or integration methods for specific applications, considering factors like the active area of smart textiles, their structure, energy consumption and the available energy that can be harvested from the environment.
Integrating and coordinating computing and sensing elements with these power sources also present challenges that need addressing. As electronic devices become smaller, more affordable, and more embedded within smart materials, managing power consumption becomes critical, especially as it increases with the number of devices. Here, low-power distributed networks gain importance, and AI plays a key role. An AI system that learns from its environment and becomes self-aware from the sensor data can manage its power efficiently, knowing when to sleep and enter low-power states or wake-up, based on the context.
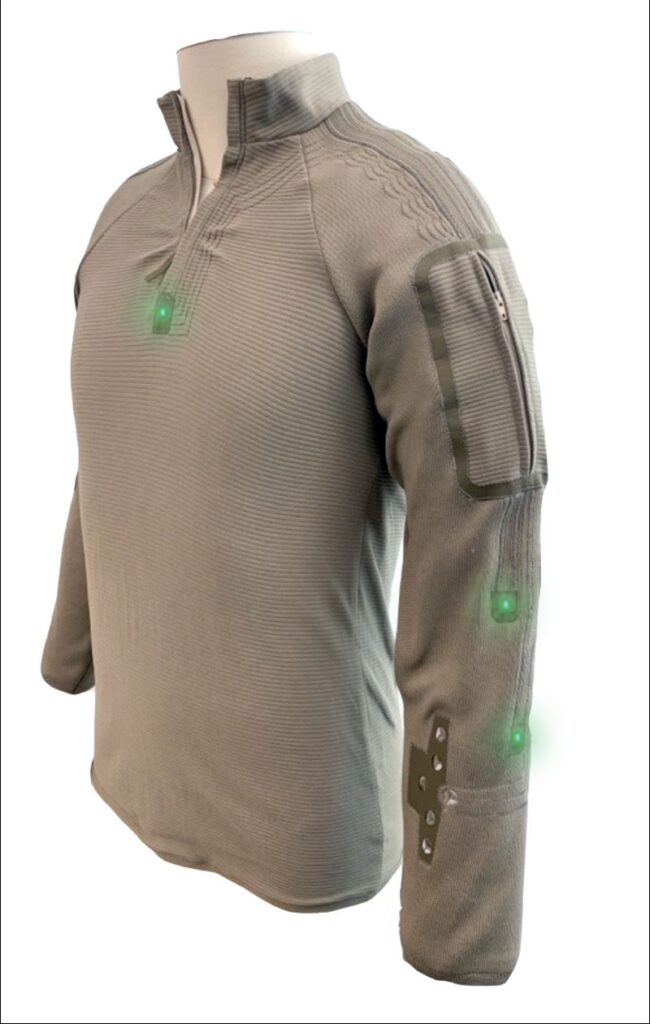
Q: If we step away from smart materials for a moment … how can AI support R&D and prototyping of other new materials that could be important in future products?
CK: I’m incredibly excited about how AI will accelerate novel material development. Identifying new materials is a complex and slow process. One of the roadblocks is identifying where to start and what new variations to experiment with. Traditional trial-and-error methods, even if well informed by prior work and informed hypotheses, are extremely time consuming.
AI has already been proven in certain applications to dramatically accelerate this process by predicting the performance of new materials based on previously collected data, optimizing material properties for specific objectives within a set of constraints, and even automating experimentation with AI-driven robotic systems that are able to autonomously test new material or structure combinations. While textile-specific applications of these methods are less common, I’m confident that, as parallel industries mature these techniques, they will rapidly become more accessible.
IW: Ergonomic comfort is crucial for functional garments worn during various activities, especially activewear and protective clothing. We recently developed the Peristaltic Suit (PS-Suit), an active pneumatic compression and physiological sensing spacesuit for intra-vehicular activities, designed to study and regulate the cardiovascular system during prolonged exposure to microgravity. The mechanical characteristics of space wear should match the degree of freedom, range of motion, force and moment of human joints. To inform our spacesuit design, we analyzed the distribution of muscle and bone tissues, blood flow, and mobility across body joints.
Other researchers at the MIT’s Human Systems Laboratory have employed similar technology using active pneumatic textiles as a soft exoskeleton. By embedding bladders into knitted pockets and sleeves around the joints, they aimed to assist astronauts in walking, climbing, and enhancing their mobility during space explorations.
With the advancement of digital fabrication and 3D fabric visualization software, which are becoming more user-friendly and powerful, we are getting quite good at using machine knitting and computational design to create data-informed, personalized clothing. I recognize the potential of AI-driven 3D generative design, taking into account various parameters such as mobility, breathability, stretchability, and radiation or temperature profiles—or even detailed visual patterns—in aiding the prototyping and development of functional textiles and garments. This approach helps to optimize aesthetics, functionality and wearability for everyday wear or for targeted applications and environments—not just for space wear, but also for diving suits, firefighter uniforms or racing suits.
Sustainable textile materials and manufacturing are also gaining interest in both research and industry, as textiles are among the world’s most polluting industries. It would be interesting to explore how AI design and additive manufacturing could facilitate the development of biodegradable or transient textile products by adjusting the product degradation rates through fiber material composition and various textile architectures.
This would particularly be useful for implantable and biomedical textiles. Additionally, it’s worth considering how we can employ “design for disassembly” or modular strategies, transforming textile products back into raw materials or their primitive components (such as fibers or chiplets) to enable reconfiguration, recycling, or reuse.
Q: What do you think is the most likely area for the use of AI to be first accepted (or widely accepted) in the textile industry?
CK: AI-enabled technologies are already being adopted in the industry in every area, from trend forecasting and demand prediction to quality control and product design. AI-trained vision systems can quickly detect defects in fabric during manufacturing. AI can be used for supply chain optimization, particularly to aid in demand forecasting and inventory management.
Among product designers, AI image generators such as DALL-E or Midjourney, as well as large language models, are already being used extensively for ideation and inspiration for new product development. However, while AI tools in the near-term are inspiring and their potential promising, they still do not come close to replacing human judgement and expertise in most applications. While AI is excellent at making suggestions and rapidly generating concepts, final decisions often still require human intervention to ensure practicality, accuracy and usefulness.
Overall, the application areas for AI in the textile industry are endless, and the development potential is exciting; however, there are many hurdles that still need to be overcome. The effectiveness of AI is dependent on the availability of extensive and high-quality datasets. Currently, the relatively low level of standardization in formatting, testing and reporting in the advanced textile space is limiting the ability to train and apply AI. That said, rapid advancements are being made, and I’m optimistic that as the quality and volume of relevant data improve, the effectiveness of these AI tools will also improve.
IW: As digital fabrication technologies, including knitting machines and textile development software, become more accessible to designers, which is currently happening, we will begin to harness AI for creating various textile patterns, textures and forms, as well as garment styles, inspired by traditional and contemporary cultures. While there are still complexities in directly translating textile designs into low-level knitting machine instructions, with more data and training, AI will become better at converting images straight into knittable programs. Our colleagues at MIT CSAIL are currently looking into this problem.
AI will also significantly help reduce prototyping time and trial-and-error efforts. Eventually, we will reach a point where we all trade and produce textile and apparel designs through digital files and on-demand manufacturing. Scanning and knitting a textile artifact would be possible at the push of a button.
Janet Preus is senior editor of Textile Technology Source. She can be reached at janet.preus@textiles.org.